
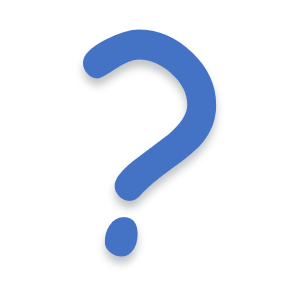
You are absolutely correct! I just couldn’t think of a way to further dive into that nuance, but I also wanted the example to be relatable and tangible. Thank you!
You are absolutely correct! I just couldn’t think of a way to further dive into that nuance, but I also wanted the example to be relatable and tangible. Thank you!
You used the phrase correctly, and your English is great!
The above commenter was rudely stating that your observation is not correct as it’s has always been this way.
This is the only response required. I’m quickly becoming exhausted of reading everyone’s epiphany on “enshittification” as if it’s some natural eventuality. Yes the money must eventually come, but not always at the expense of platform quality. If anything the results we see from “enshittification” are due to the fact that most businesses fail eventually due to poor leadership.
Just to echo what you have already said, money today is simply more expensive than it used to be. We even see the impacts of macro monetary decisions on households.
Buying a house or a car on loan is far more expensive than it would have been a year and half ago. A $500,000 house in 2021 would cost $2,000 a month at 2.75% interest and 20% down. Today same that payment is $2,800 or 40% more expensive at 7.75% interest.
Modern companies live on revolving debt, so if their suddenly gets 40% more expensive and that same amount of money is also less valuable at the same time (inflation), then they need to make up the difference somehow.
Corporations are trying to find the balance between squeezing more revenue to pay their ever increasing debt bills while also not destroying the environment that attracted the users (their products) in the first place. Twitter and Reddit are just going about it horrifically because of poor business leadership and decision making. Netflix’s approach appears to be sustainable, and there is no doubt that YouTube will be fine in the long run.
This is not meant to be apologetic to the decisions made by Twitter and Reddit. They’ve made their bed through their own horrible decisions, and now they’ve got to sleep in it.
much less serious
EXCEPT when it came to grammar and spelling! Honestly, I miss that kind of pedantry from the old days.
I am an LLM researcher at MIT, and hopefully this will help.
As others have answered, LLMs have only learned the ability to autocomplete given some input, known as the prompt. Functionally, the model is strictly predicting the probability of the next word+, called tokens, with some randomness injected so the output isn’t exactly the same for any given prompt.
The probability of the next word comes from what was in the model’s training data, in combination with a very complex mathematical method to compute the impact of all previous words with every other previous word and with the new predicted word, called self-attention, but you can think of this like a computed relatedness factor.
This relatedness factor is very computationally expensive and grows exponentially, so models are limited by how many previous words can be used to compute relatedness. This limitation is called the Context Window. The recent breakthroughs in LLMs come from the use of very large context windows to learn the relationships of as many words as possible.
This process of predicting the next word is repeated iteratively until a special stop token is generated, which tells the model go stop generating more words. So literally, the models builds entire responses one word at a time from left to right.
Because all future words are predicated on the previously stated words in either the prompt or subsequent generated words, it becomes impossible to apply even the most basic logical concepts, unless all the components required are present in the prompt or have somehow serendipitously been stated by the model in its generated response.
This is also why LLMs tend to work better when you ask them to work out all the steps of a problem instead of jumping to a conclusion, and why the best models tend to rely on extremely verbose answers to give you the simple piece of information you were looking for.
From this fundamental understanding, hopefully you can now reason the LLM limitations in factual understanding as well. For instance, if a given fact was never mentioned in the training data, or an answer simply doesn’t exist, the model will make it up, inferring the next most likely word to create a plausible sounding statement. Essentially, the model has been faking language understanding so much, that even when the model has no factual basis for an answer, it can easily trick a unwitting human into believing the answer to be correct.
—-
+more specifically these words are tokens which usually contain some smaller part of a word. For instance,
understand
andable
would be represented as two tokens that when put together would become the wordunderstandable
.