
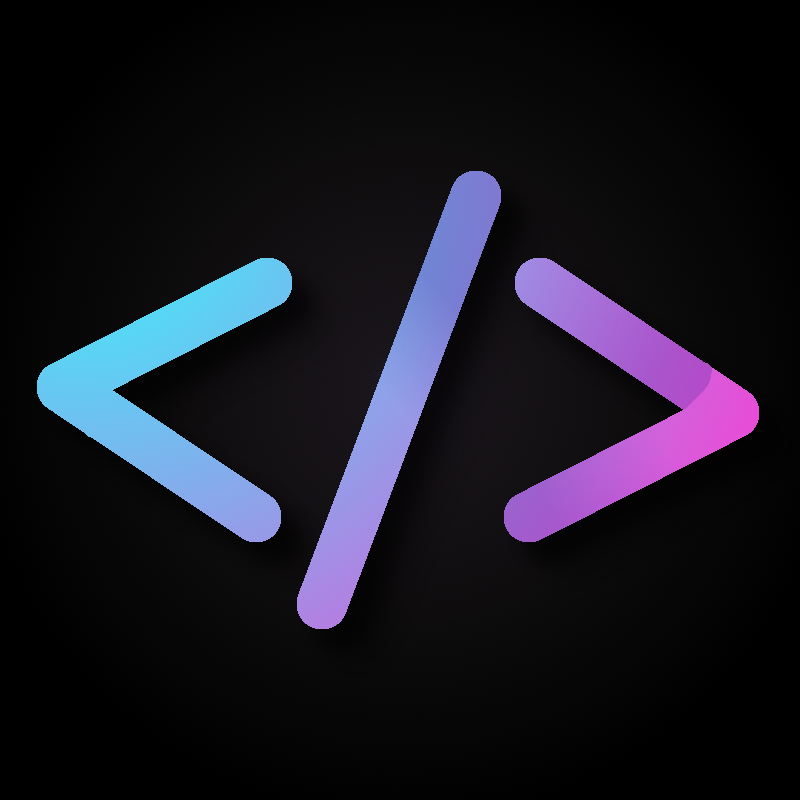
I somehow didn’t think a regular JIT solution might be applicable here, but it is. Thank you! There seems to be a number of projects doing JIT for C++, will look at them.
I somehow didn’t think a regular JIT solution might be applicable here, but it is. Thank you! There seems to be a number of projects doing JIT for C++, will look at them.
So far I’ve been following recommendations from this person: https://old.reddit.com/r/NewMaxx/comments/16xhbi5/ssd_guides_resources_ssd_help_post_your_questions/
Kernel is not a monolithic application, and you cannot develop it like one. There are tons of actors: independent developers, small support companies (like Collabora), corporations, all with different priorities. There is a large number of independent forks (e.g. for obscure devices), that will never be merged, but need to merge e.g. security patches from the mainline. A single project management tool won’t do, not your typical business grade tracking&reporting tool.
CI is already there. Not a central one—again, distributed across different organizations. Different organizations have different needs for CI, e.g. supporting weird architectures that they need to develop against.
There is a reason Torvalds created git—existing tools just wouldn’t work. There might be a place for a similar revolution regarding a bugtracker…
This plea for help is specifically for non-coding, but still deeply technical work.
The thread is an attempt to merge a new file system, bcachefs
. This is a large change, requiring a lot of review from experienced developers, and getting anyone to do this work turned out to be difficult. Darrick here started talking how, in general, all development of file systems in Linux is troubled by lack of manpower.
I guess the best start would be to have a person to organize volunteers.
Another idea that just occurred to me. Maybe position: absolute; both the real content and the gibberish content with the same top, left, width, and height attributes so that the real content and the gibberish overlap and occupy the same location on the page. Make sure both the real and gibberish content elements have no background so that remains clear. Put the gibberish content in the DOM before the real content. (I think that will ensure that the gibberish appears behind the real content even without setting the z-index.) And then make JS set the color of the text in the gibberish element the same color as the background so humans can’t see it.
Be aware that these techniques can affect accessibility for people using screen readers.
As of May 2023, 65% of the Ukrainian refugees that left Ukraine starting February 2022 and decided to stay in Poland found a job—so, within around a year, as opposed to 5-6 years as in the article. Cultural similarity here is likely making it much, much simpler. For those who want to read more about the situation of Ukrainian refugees in Poland, this report by Polish National Bank (Narodowy Bank Polski, NBP) might be useful: https://nbp.pl/wp-content/uploads/2023/05/Raport_Imigranci_EN.pdf (in English!), there is a lot of interesting details.
Yep, thank you, that’s pretty close to what I imagined!
A lack of planning on your part doesn’t constitute an emergency on mine.
Though I kind of think Japanese grammar cannot express this thought and the closest you can get is Ganbatte!
Good question! I quickly found this table, though this is yearly statistics only: https://www150.statcan.gc.ca/t1/tbl1/en/tv.action?pid=3510019201
Will they keep the dense email list view as an option? Seeing more than the 14 email messages visible on the screenshot in the post is useful to sort out large folders.
Last job, we started writing mixing bits of Kotlin in an otherwise mostly-Java in a monolithic Spring-based service. Good experience.
One reason (among many) is that employment in American companies is less stable than in Europe with strong employment laws. Twitter could not do the same type of layoffs in Europe, with stories like this one being pretty common. But this safety net has a cost, and the cost is a part of total employment cost for employers. Whether the safety net is worth it for employees in IT, that’s another matter—but it can’t not be taken into account because of the law.
BTW, in some European countries there is a strong culture of IT workers doing long-term contractor work exactly to trade off employment laws for (usually quite a lot) higher wage.
I found it crazy useful to study old, established, mature technologies, like relational databases, storage, low-level networking stack, optimizing compilers, etc. Much more valuable than learning the fad of the year. For example, consider studying internals of Postgresql if you’re using it.
Given these criteria, ggplot2 wins by a landslide. The API, thanks to R’s nonstandard evaluation feature, is crazy good compared to whatever is available in Python. Not having to use numpy/pandas as inputs is a bonus as well, somehow pandas managed to duplicate many bad features of R’s data frame and introduce its own inconsistences, without providing many of the good features¹. Styling defaults are decent, definitely much better than matplotlib’s, and it’s much easier to consistently apply custom styling. Future of ggplot2 is defined by downstream libraries, ggplot2 is just the core of the ecosystem, which, at this point, is mature and stable. Matplotlib’s activity is mostly because that lack of nonstandard evaluation makes it more cumbersome to implement flexible APIs, and so it just takes more work. Both have very minimal support for interactive and web, it’s easier to just use shiny/dask to wrap them than to force them alone to do web/interactive stuff. Which, btw, again I’d say shiny » dask if nothing but for R’s nonstandard evaluation feature.
Note though that learning proper R takes time, and if you don’t know it yet, you will underestimate time necessary to get friendly. Nonstandard evaluation alone is so nonstandard that it gives headaches to people who’d otherwise be skilled programmers already. matplotlib would hugely win by flexibility, which you apparently don’t need—but there’s always that one tiny tweak you would wish to be able to do. Also, it’s usually much easier to use the platform’s default, whatever publishing platform you’re going to use.
As for me, if I have choice, I’m picking ggplot2 as a default. So far it was good enough for significant majority of my academic and professional work.
¹ Admitably numpy was not designed for data analysis directly, and pandas has some nice features missing from R’s data frames.
At such scale, a scraper wouldn’t be necessary, that’s easily doable by humans involved in these communities—with a human touch as well.
Yes, many times. And I recall using the technique manually back when I was working with Subversion many, many years ago. No fun stories though, sorry, it’s just another tool in a toolbox.
Storj does it at 7 USD/TB. And there are providers that technically provide unlimited bandwidth, like Hetzner’s dedicated servers; they still have some abuse limits, but even working within the limits should make it much cheaper. This means custom engineering though.
I’d probably be fine with hundreds or thousands of these hanging in memory. I suspect the generated code for a single query would be in hundreds of kilobytes, maybe a megabyte. But yeah, this is one of those technical details I’d worry about.
Not sure how a HTTP server would solve the CPU bottleneck of scanning terabytes of data per query?